AI has exploded in the last year and it feels like new, groundbreaking tools are coming out daily. Obviously, we are very interested in how AI tools will impact the UX research industry, especially things like automated surveys, video coders and GPTs that will turn interview transcripts into polished executive summaries.
AI is becoming a popular research tool because it’s quick. Really quick. But is it the research silver bullet that it’s being made out to be, or are there potential pitfalls to using machines to understand human behaviour?
Where AI and UX Research meet
*Things change every day, so this is just a snapshot of where we are today, but it might not be the same tomorrow.
It’s quick, it (can be) cheap and it sounds super smart. The potential is huge to reduce the time taken by some of the longer tasks in the research process.
However, as with all things in research, the how and the why you use something needs to be carefully considered.
✅ AI works really well as a starting point for question-writing
Asking an AI tool to write a list of research questions or a recruitment screener from loose requirements is surprisingly effective. And fast! Not excellent, but definitely “junior researcher” level stuff.
For example, when asked to “write me research questions for finding out the user experience of a banking app”, this tool gave solid recommendations.
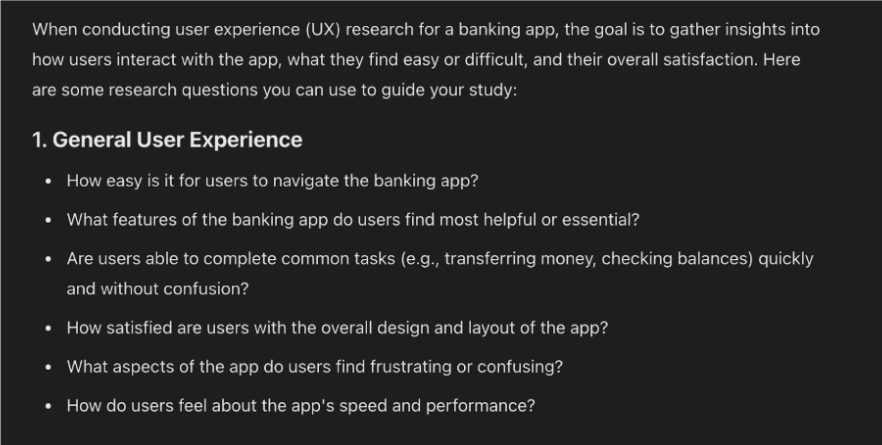
Although these are a good starting point the questions are a little too broad and may yield unhelpful or misleading data. For example, what features users find most helpful is not the same as what may be essential. Being able to complete a task quickly is not the same as completing one without confusion.
As we can see, these are not the actual questions we’d ask a respondent, but they’re a good start to point you in the right direction. Time will be needed to refine these to ensure they capture what the client really wants to know.
⚠️ Be wary of ‘Garbage in garbage out’
It’s a well-known fact in research that asking the wrong questions will give the wrong data.
Knowing the right thing to ask and evaluating if your line of questioning is going to get you the answers you need is often why we’re hired in the first place. Not to know what to ask, but what is the best thing to ask, and when. Read more about this in Why we need to know the business of our client. As researchers, we know the power of letting the users direct the answer.
We’re big fans of scenario-based tasks and critical incidents
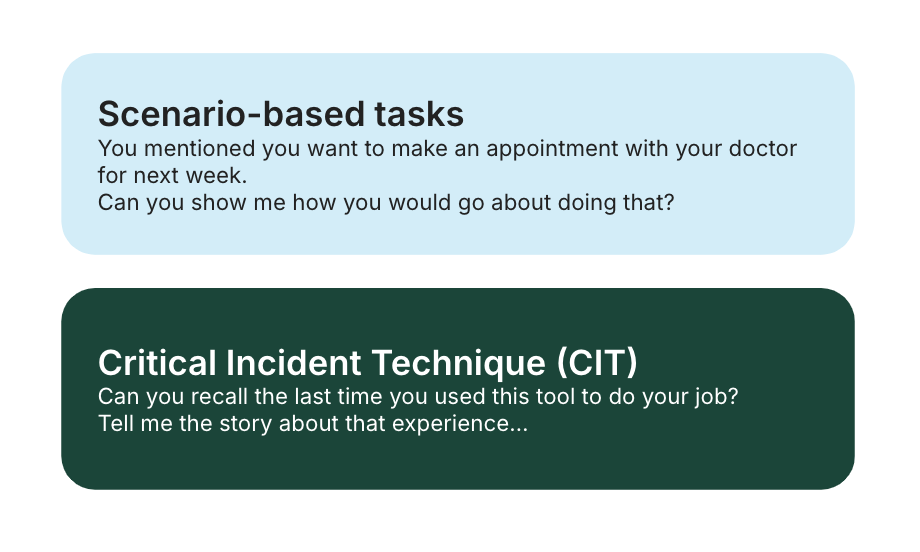
Instead of leading them with a question like, “How easily did you navigate the banking app” we’d rather ask, “Tell me about what just happened” or, “Tell me about the last time you did something like this”. This helps users tell us what really happened, not just what they think we might want to know.
✅ AI undeniably excels at analysis
AI can connect the dots between lots of pieces of information at a speed that is frankly impossible for humans to do. After all, pattern recognition and language learning is what it was built for.
What this means for research is that it can be fed interview transcripts (sometimes even the raw interview recordings), rough notes and doodled ideas to generate high level summaries instantly. This is massive in rapidly shaping an initial picture of the research and identifying the biggest themes.
⚠️ However, we’ve seen AI struggle with sentiment
While AI is great at crunching volumes of data, it can struggle with complex inputs. It particularly struggles to understand sentiment (what people feel about something) from the subtle shifts in a participant’s body language or tone that a human researcher may pay attention to.
AI struggles to figure out why something is happening if it’s not said out loud. A researcher is able to apply the participants’ context, mental models, previous experiences, and personalities to their actions and understand their behaviour. We absorb these data points intuitively as we’re humans ourselves.
It’s important to remember that research is not just about connecting the dots, it’s about understanding them too.
We’re cautious about using AI for synthesis
Analysis is finding the pattern in the facts (through matching like-for-like) but synthesis is knowing what the pattern means. We’re still sceptical of AI-generated reports as they stop at analysis, and the insights can be a bit too obvious. Just stating the facts doesn’t tell you why they’re important.
We’ve seen it make a mountain out of a molehill
AI tends to over-index on certain insights because they were said many times. However, we know that a fact can be repeated a lot, but not be super critical to the problem we’re investigating. AI struggles to make that distinction and will present it as a major theme in the analysis, potentially skewing the interpretation of a problem area.
AI may not get down to the “why” of the problem
An AI generated report might miss the “so what does this mean for you” layer of interpretation. Synthesis normally includes an understanding of the bigger picture of the research, taking the client context into consideration when looking at the facts. This is why the human researcher needs to be familiar with the data and not only use what AI said. It’s up to the researcher to deliver an understanding of what happened and why it’s important by translating the data’s value for the client.
✅ We think AI is best Paired with a human
As the common trope goes, AI will not replace humans, but humans using AI will replace those who don’t. In research, we think AI works better as a research aid, doing the legwork and acting as an evaluator more than a creator.
Ask it to check your questions
AI is great at making questions more direct or softening them for a sensitive subject matter by rewriting them for you.
For example, are they missing an important aspect of your research focus? Could they be asked in a less confusing way? Could they be softened to better suit a sensitive subject matter.
Ask AI what you might have missed in your report
Use AI to cross check your own interpretation. This way you can avoid over-indexing on one insight that may not be the most important thing the client wants to know, but may pick up an angle on the data you hadn’t noticed.
Editing report writing
Make your writing easier to understand without the lengthy editing process. Asking an AI tool to help me rewrite an insight in a more direct way has transformed how I think about delivering information in the first place.
Choose your weapons and go forth!
At the end of the day, it’s important to remember that AI tools are still evolving. Discourses around data security, accuracy and privacy are shaping the way people see and use AI tools.
Experiment with AI and see where it augments your workflow best. Keep in mind that security is an important consideration when managing proprietary data, so check how your inputs might be used by the AI to train its own model first.
And finally, by the time you read this, the AI landscape may have changed entirely. It would be remiss to completely discard the value it has for our industry when it’s still growing, but with all things, we believe moderation and caution is the key!